Generative AI defined
Generative AI, one type of artificial intelligence, is rapidly transforming our familiar educational environments with the promise of dynamic, personalized, and exciting learning and teaching experiences, while also raising concerns about authenticity in our work, its impact on the environment, and uncertainty about how it is changing the world around us
Generative AI refers to any system capable of creating (i.e., generating) new content, such as text, images, and music, by learning from vast amounts of training data. Because they are so easy to use, large language models (LLMs) are impacting the day-to-day work of students, teachers, scholars and others at Tufts. LLMs, such as ChatGPT and Claude, generate human-like text responses based on vast training datasets. While LLMs specialize in text, other AI models, trained on different data types, can generate images, music, video, and more from simple prompts.
If you want to learn more, this animated video is a fun introduction to generative AI addressing: what it is, where it comes from and where we are going.
It is important to recognize generative AI tools from simple automation tools that assist with tasks to more advanced models that can create things using text, voice, images and video. These systems are also embedded in many of the applications we use daily—ranging from web platforms like Canvas to the software on our phones and tablets. Increasingly, they appear in our workflows, whether through internet searches or document readers like Adobe.As we navigate this changing terrain, we need to make decisions about how to use these tools effectively and ethically, and to reflect on how they are impacting our experience and journey as a learner, teacher or administrator.
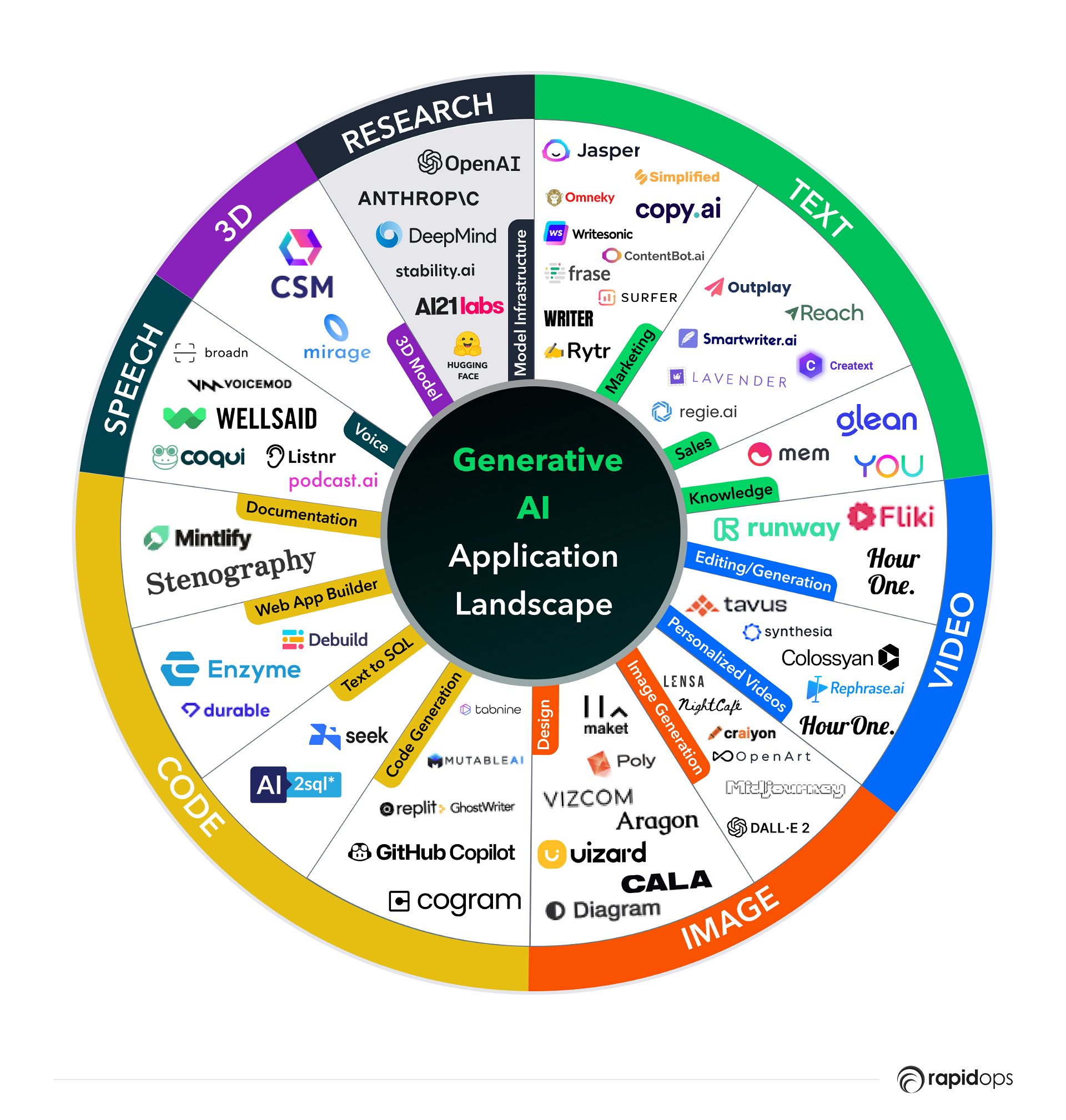
Applications in Education:
- For Faculty: Enhancing content delivery, automating grading, and personalizing instruction.
- For Students: Assisting with writing, summarization, research, and problem-solving.
- For Administration: Automating routine tasks and enhancing decision-making processes.
How does Generative AI work?
Can I ask AI for medical advice? Will it give me useful references for my paper? When is AI a trusted editor for my writing?
Because Generative AI responds to any question we ask, understanding how it works is essential for making informed decisions about when and how it can be useful
Many users rely on AI to provide facts or data, only to be frustrated when the response is inaccurate or completely fabricated—a phenomenon known as “hallucination.” Since AI doesn’t “retrieve” knowledge like a search engine, but instead generates text based on patterns in its training data, we can’t rely on the accuracy or truth of its confidence response. Given that, one might find it more surprising that AI’s answers are often correct, simply because statistical patterns in its input data often align with reality. However, when using AI it’s critical to recognize that AI does not fact-check, verify sources, or “know” truth from falsehood; it merely predicts the most probable sequence of words in a response.
Similarly, AI does not form a conceptual model of the real world—it generates text and images based on learned statistical relationships rather than structured reasoning. Since its vast training datasets include biased and sometimes inaccurate data from various online sources (Washington Post: What AI Chatbots Are Learning), it can reinforce misinformation rather than correct it.
Another thing that often surprises users is that the same prompt can yield vastly different responses depending on which AI tool is used and what context the model is drawing from. This variation occurs because AI doesn’t develop an idea conceptually before responding—it generates text token by token, predicting the next word based on probabilities in its training data. Differences in model architectures, training data, and tuning parameters also contribute to these variations in response (See this visual explainer for more details). Users can also shape the responses by providing context within a conversation. For example, if you share an article or notes before asking a question, the AI will adjust its predictions based on that additional input, increasing the likelihood that related terms and concepts appear in the response. Some AI chatbots even have a form of persistent memory, allowing them to retain information from past interactions across multiple sessions.
Why should I care about this?
Because AI isn’t all knowing, it’s a tool not the truth. The model is structured in such a way that it is literally trying to predict the next word, it is not algorithmic. We must acknowledge this so we are not taking what it gives us at face value. We need to reflect…
AI, especially generative AI, is not a fact machine—it doesn’t pull direct answers from a database like search engines like Google do. Instead, it creates responses based on patterns in the data it has been trained on.
We need to push ourselves to critically think when interacting with AI-generated content and how it is helping us. Everything is not web enabled and is part of a framework that predicts and has its own biases that we must take into account.
AI doesn’t “understand” the way humans do. It generates text, images, or ideas based on what it has learned. Think of it like auto-complete on your phone—it predicts what comes next based on context
https://ig.ft.com/generative-ai/