Think Critically, Not Just Quickly – Using AI Without Losing Learning
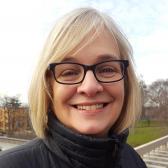
An Interview with Jennifer Ferguson, Head of User Experience & Student Success at Tufts Tisch Library
By Mehek Vora
A librarian, educator, and former private equity research analyst, Jennifer Ferguson has been at the forefront of AI literacy, teaching students and faculty how to critically engage with these ever-evolving tools. Jennifer’s journey with AI isn’t one of mere curiosity—it is intertwined with her professional experiences. Before stepping into the world of academia, she worked as a research analyst in private equity, analyzing tech startups and emerging technologies. She’s seen AI grow from niche applications in the early 2000s to the all-encompassing, algorithm-driven world we live in today and recognizes “AI isn’t a new revolution—it’s the next step in a long evolution.” As a librarian, she views AI as an extension of a long-standing challenge: How do we teach people to evaluate information in an age where algorithms filter what we see and we don’t always know where the data is coming from?
Teaching AI Literacy at the Library (link to their website/canvas/resources)
Jennifer and her team at Tisch Library are teaching students not only how to use AI for research but also to critically evaluate its limitations and ethical implications. Through a mix of synchronous and asynchronous learning modules, students engage with technical topics like algorithmic bias and AI hallucinations to understand where the information is coming from. It helps break the stereotype of thinking about robots when hearing ‘artificial intelligence’. As she emphasized in her interview, it’s important that all students understand:
- “AI is a predictive algorithm working across enormous data sets. Large Language models aren’t sources of absolute truth—they’re just really good at predicting what sounds right. AI isn’t thoughtfully crafting responses; it’s playing an advanced game of autocomplete, guessing the next best word based on the data it has.”
- “This becomes a real problem in research if students trust the tool, only to realize later that the article they’re looking for was never published—it was just a convincing hallucination. The issue isn’t just misinformation; it’s the illusion of credibility. AI can sound confident, but a polished answer isn’t always a correct one—and that’s exactly why individuals need to approach it with a healthy dose of skepticism and ability to critically evaluate what they are working on. “
Can AI streamline time-consuming tasks at Tisch Library?
Jennifer and her team have been exploring ways to integrate AI into library workflows to free up more time for deeper, more meaningful work.
Using Microsoft Copilot agents, the library team trains AI with their own data, ensuring that it understands their unique needs and materials. This approach allows them to automate the process of generating descriptions for library resources—a massive undertaking given that their website houses over 500 different items. Jennifer tested the system on a small dataset of 27 items, and the results were promising—the AI produced high-quality descriptions with impressive accuracy. By leveraging AI as a partner rather than a replacement, Jennifer and her team are proving that technology, when used thoughtfully, can empower libraries to serve students and faculty more efficiently than ever before.
What are we losing when we let AI do too much thinking for us?
Using AI to produce polished work without actually engaging with the material is not just about plagiarism or academic dishonesty. The deeper issue is metacognition—the ability to think about one’s own learning. If AI is doing the heavy lifting, are students truly understanding concepts, or just submitting well-structured AI-generated answers? We see an increasing use of education for credentialization – getting a credential rather than “I need to learn how to think.”
That’s the risk AI poses—it’s quietly replacing essential cognitive skills, and students may not even realize what they’re losing (i.e. you don’t know what you don’t know). The challenge for educators, then, is not just how to incorporate AI into learning, but how to ensure that learning still happens. Jennifer offers a simple but powerful analogy:
“I already know how to read a map. But if I had never learned and started relying only on GPS, my brain wouldn’t develop that spatial awareness. I wouldn’t even know that I was missing it.”
Jennifer doesn’t advocate for banning AI—far from it. Instead, she urges a shift toward AI literacy with a focus on understanding the power imbalance. The idea that there is a gap between AI model training data that users are not aware of but engage with. It is important to be critical of AI-generated information because if you’re not an expert in a topic, you may not even recognize what’s missing. AI tools already shape our search engines, academic databases, and even job applications. Ignoring them isn’t the answer—using them wisely is.
“Everyone is already using AI—it’s embedded in everything. Ignoring it won’t make it go away. But we can teach people how to make good choices about it.”
As AI continues to evolve, the real challenge isn’t whether students and educators will use it—it’s whether they’ll use it thoughtfully, critically, and ethically.
Jennifer leaves us with the question: “What are we losing when we let AI do too much thinking for us?”