Ph.D. student specializing in Nutrition Interventions, Communications, and Behavior Change at Tufts Friedman School of Nutrition Science and Policy.
With over a decade of diverse healthcare experience spanning long-term care facilities, acute care hospitals, consulting firms, and life sciences companies, Marcia brings a profound firsthand understanding of the multifaceted challenges that impact health. She is committed to leveraging her practical experiences and expertise to drive equitable and sustainable transformations within healthcare systems and communities.
Her research interests explore the intersection of diet-related diseases and the socio-political determinants that contribute to nutrition and health inequity. She strives to conduct research that informs policy, program design, and practice guidelines to address the upstream drivers of health inequity, applying behavior change science to develop effective interventions. Marcia holds an MBA from Texas Woman’s University and a BS in Dietetics from Michigan State University.
Final Presentation
Final Project
AI-Powered Thematic Analysis: Improving Accuracy and Efficiency in Qualitative Health Behavior Research
Project Description
Abstract
This study aims to enhance qualitative data analysis of health behavior data by identifying efficient and accurate AI models for thematic analysis and coding of unstructured interview data. Leveraging natural language processing (NLP) techniques, we will evaluate three individual, and one ensemble AI model to determine the most precise and comprehensive approach for qualitative data analysis. Each model’s performance will be benchmarked against traditional qualitative data analysis conducted by trained researchers to assess accuracy and efficiency. We hypothesize that the combined AI model will outperform the individual models in thematic coding accuracy and comprehensiveness. Our findings will demonstrate the potential of NLP to optimize qualitative research, enabling greater insights from larger datasets while alleviating the time and resource burdens typically associated with qualitative analysis. This research aims to pave the way for more scalable and detailed qualitative studies.
Introduction and Rationale
Qualitative research captures detailed, non-numerical data through open-ended questions, providing rich insights across human and social sciences (Moser & Korstjens, 2017). Traditional methods like focus groups and in-depth interviews reveal participants’ perspectives but face significant challenges in analyzing large volumes of unstructured data (Guetterman et al., 2018). Manual thematic analysis can be labor-intensive, and time-consuming, which limits the depth of analysis in large-scale studies.
Artificial intelligence (AI) like natural language processing (NLP) offers promising solutions to these challenges by automating the thematic coding process, enhancing efficiency, consistency, and scalability. Despite these capabilities, the integration of NLP in qualitative research remains underexplored, particularly regarding the identification of the most effective models for thematic analysis in health behavior qualitative research.
This study aims to address this gap by systematically evaluating NLP techniques for their effectiveness in thematic analysis and coding of unstructured interview data. We will compare these models to traditional qualitative analysis conducted by trained researchers to identify the most accurate and comprehensive approaches. This research is significant for several reasons:
- Efficiency and Consistency: AI models can process large datasets quickly and consistently, reducing the time and resources required for analysis.
- Scalability: AI-driven approaches enable the analysis of larger sample sizes, providing more robust and generalizable findings.
- Enhanced Insights: Automated analysis can uncover patterns and themes that might be missed by human analysis alone, leading to deeper and more comprehensive insights.
By demonstrating the feasibility and advantages of using NLP for qualitative health behavior data analysis, this study aims to streamline qualitative research, enabling researchers to handle larger datasets without compromising the quality of insights. Identifying the most effective AI models for thematic analysis will support the broader adoption of these technologies, enhancing the overall quality and impact of qualitative studies.
Literature Review
This review explores the trends, challenges, and opportunities in applying NLP to qualitative data in health behavior research. Nawab et al. (2020) showcased NLP’s capability to identify themes in public health studies, whereas Van Buchem et al. (2022) utilized sentiment analysis and topic modeling to assess patient perspectives on healthcare improvements. However, challenges related to data quality and preprocessing were also highlighted by Van Buchem et al. (2022). Data processes that structure formatting and correct spelling prior to analysis can be critical to NLP application effectiveness. Additionally, the lack of transparency and interpretability in some NLP models, noted by Rudin (2019), complicates bias detection and mitigation efforts. Guetterman et al. (2018) also cautioned that NLP techniques may overlook subtle contextual nuances inherent in qualitative data.
Despite these challenges, NLP presents substantial opportunities for advancing behavioral health research. It can streamline qualitative data analysis, improving efficiency in thematic and sentiment analysis to reveal meaningful patterns in patient narratives.
Use Case Description
Objective: The objective of this study is to identify the most accurate and comprehensive natural language processing techniques for thematic analysis and coding of health behavior qualitative interview data.
Methodology/Approach: Unstructured interview data on health behaviors will be collected from a diverse sample, cleaned, preprocessed, and anonymized for privacy. Three individual AI models and one ensemble model will be selected for thematic analysis. NLP techniques, such as topic modeling and sentiment analysis, will be applied, with text processing standardizing the data. The AI models will be trained using cross-validation and evaluated on accuracy, comprehensiveness, and efficiency, compared to traditional qualitative analysis. Benchmarking will ensure reliability, and hypothesis testing will determine if the ensemble model outperforms the individual models. Scalability and resource optimization will be assessed on larger datasets. Findings will be presented to demonstrate the potential of AI and NLP in optimizing qualitative research, with implications for health behavior research discussed and disseminated through publications, conferences, and workshops.
Key Stakeholders: Researchers in qualitative data analysis, healthcare professionals, policy makers, technology developers specializing in natural language processing (NLP), study participants.
Proposed AI Techniques/Tools: We will evaluate three individual NLP techniques, these include Bi-directional Encoder Representations from Transformers (BERT), Traditional Machine Learning (ML) models, and Word Embedding Models. Then we will develop an ensemble model, using the top preforming NLP techniques to evaluate its effectiveness compared to using an individual model and traditional qualitative research data analysis methods.
Knowledge Graph

Edges (Relationships)
- AI Models → uses → NLP Techniques
- NLP Techniques → analyze → Qualitative Data
- Traditional Qualitative Analysis Methods → benchmark → AI Models
- Health Behavior Research → studies → Health Behavior Influences/Factors
- Health Behavior Influences/Factors → shape → Qualitative Data
- Health Behavior Research → designs → Health Behavior Interventions
- Health Behavior Interventions → target → Health Behavior Influences/Factors
- Health Behavior Research → produces → Research Outcomes
- Ethical Considerations → guide → Handling of Qualitative Data
- Research Outcomes → inform → Health Behavior Interventions
- Researchers → conduct → Health Behavior Research
- Participants → provide → Qualitative Data
- Researchers → implement → Health Behavior Interventions
- Behavior Change → can result from → Health Behavior Interventions
- Data Quality → affects → Research Outcomes
- Data Quality → affects → AI Models
- Socioeconomic Factors → affect → Health Behavior Influences/Factors
- Environmental Factors → affect → Health Behavior Influences/Factors
- Policy Makers → influence → social economic and environmental factors
Conceptual Diagram:
Relationships:
- AI Models influence the application of NLP Techniques.
- NLP Techniques enhance the processing of Qualitative Data.
- Qualitative Data undergo Thematic Analysis.
- Thematic Analysis results are compared against Traditional Qualitative Analysis Methods.
- Health Behavior Research provides the source of Qualitative Data.
- AI Models and NLP Techniques reduce Researcher Effort.
- Reduced Researcher Effort leads to greater Efficiency and Scalability.
- Improved Thematic Analysis contributes to deeper Insight Depth.
Summary:
The proposed solution, leveraging AI models and NLP techniques, aims to enhance the efficiency, scalability, and depth of qualitative data analysis in health behavior research. By reducing researcher effort and enabling more comprehensive thematic analysis, the solution provides a robust framework for generating valuable insights and informing effective health interventions. Benchmarking against traditional methods ensures the reliability and accuracy of the AI-enhanced approach, paving the way for broader applications across various research domains.
Concept Map for Practice/Policy Change:
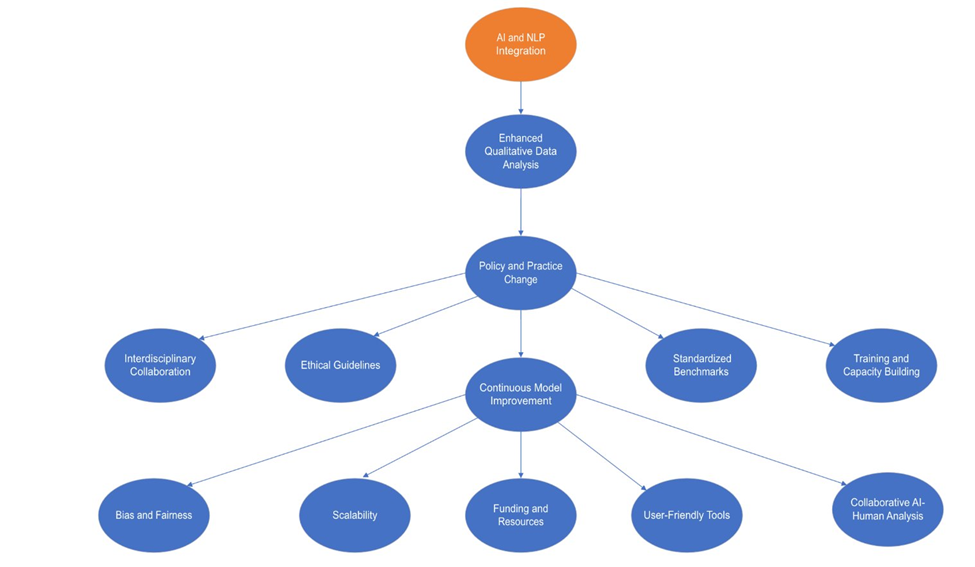
Summary:
The integration of AI and NLP can enhance qualitative data analysis, leading to more efficient, comprehensive, and scalable research. This improvement could drive policy and practice changes by providing deeper insights and reliable data. Interdisciplinary collaboration, ethical guidelines, and standardized benchmarks would support these changes, ensuring diverse perspectives and responsible AI use. Training and capacity-building initiatives would enable effective AI and NLP tool usage, while continuous model improvement would address evolving needs and mitigate biases. Adequate funding and resources will be crucial for developing and maintaining these technologies. User-friendly tools and collaborative AI-human analysis could further enhance the quality and adoption of qualitative research, fostering significant advancements in policy and practice
Ethical Considerations
Informed Consent: Informed consent should address AI techniques, data analysis methods, data protection, voluntary participation, right to withdraw, potential risks and benefits, and future data use. Developing clear, plain-language consent forms detailing the study’s purpose, methods, and data protection measures are important.
Data Privacy and Security: Handling unstructured interview data involves sensitive information, making data privacy and security critical. To address these concerns, we will anonymize all participant data, use robust encryption for data and implement strict access controls to ensure only authorized personnel can access the data.
Bias and Fairness: AI models can inherit biases from training data, leading to unfair outcomes. To mitigate this, we will use diverse and representative datasets, regularly test models for bias, and apply techniques such as reweighting and adversarial debiasing. Transparency in AI model development will be maintained to foster trust among stakeholders.
Potential Risks: AI-generated results might be misinterpreted, leading to incorrect conclusions. Additionally, over-reliance on AI could diminish the importance of human judgment. To counter these risks, we will validate AI-generated insights with traditional qualitative analysis by experienced researchers. We will also promote a balanced approach where AI augments rather than replaces human analysis.
By addressing these ethical concerns, the study aims to responsibly leverage AI and NLP techniques, enhancing the quality and efficiency of qualitative data analysis in health behavior research while safeguarding participant rights and data integrity.
Conclusion and Recommendations
The study explores the potential of AI and Natural Language Processing (NLP) in enhancing qualitative data analysis in health behavior research. Combined AI models are anticipated to improve accuracy and efficiency in thematic analysis and coding of unstructured interview data. NLP techniques can reduce time and resource burdens associated with traditional methods, facilitating more scalable and detailed studies from larger datasets.
Recommendations include integrating AI models to augment human analysis, continuously mitigating biases, and promoting transparency in AI development and interpretation. Policy engagement is advocated to establish ethical guidelines address data privacy, security, and fairness in AI-driven research.
Continual challenges relate to variability in data quality, leveraging diverse and representative datasets, and the potential oversight of contextual nuances by AI models. Additionally, bias detection and mitigation methods require further refinement to ensure fairness.
References
- Fang, C., Markuzon, N., Patel, N., & Rueda, J.-D. (2022). Natural language processing for automated classification of qualitative data from interviews of patients with cancer. Value in Health, 25(12), 1995–2002. https://doi.org/10.1016/j.jval.2022.06.004
- Guetterman, T. C., Chang, T., DeJonckheere, M., Basu, T., Scruggs, E., & Vydiswaran, V. G. V. (2018a). Augmenting qualitative text analysis with natural language processing: Methodological study. Journal of Medical Internet Research, 20(6), e9702. https://doi.org/10.2196/jmir.9702
- Guetterman, T. C., Chang, T., DeJonckheere, M., Basu, T., Scruggs, E., & Vydiswaran, V. G. V. (2018b). Augmenting qualitative text analysis with natural language processing: Methodological study. Journal of Medical Internet Research, 20(6), e9702. https://doi.org/10.2196/jmir.9702
- Kauh, T. J., Read, J. G., & Scheitler, A. J. (2021). The critical role of racial/ethnic data disaggregation for health equity. Population Research and Policy Review, 40(1), 1–7. https://doi.org/10.1007/s11113-020-09631-6
- Moser, A., & Korstjens, I. (2017). Series: Practical guidance to qualitative research. Part 1: Introduction. European Journal of General Practice, 23(1), 271–273. https://doi.org/10.1080/13814788.2017.1375093
- Nawab, K., Ramsey, G., & Schreiber, R. (2020). Natural language processing to extract meaningful information from patient experience feedback. Applied Clinical Informatics, 11(02), 242–252. https://doi.org/10.1055/s-0040-1708049
- Pillai, M., Griffin, A. C., Kronk, C. A., & McCall, T. (2023). Toward community-based natural language processing (Cbnlp): Cocreating with communities. Journal of Medical Internet Research, 25, e48498. https://doi.org/10.2196/48498
- Rudin, C. (2019). Stop explaining black box machine learning models for high stakes decisions and use interpretable models instead. Nature Machine Intelligence, 1(5), 206–215. https://doi.org/10.1038/s42256-019-0048-x
- van Buchem, M. M., Neve, O. M., Kant, I. M. J., Steyerberg, E. W., Boosman, H., & Hensen, E. F. (2022). Analyzing patient experiences using natural language processing: Development and validation of the artificial intelligence patient reported experience measure (Ai-prem). BMC Medical Informatics and Decision Making, 22, 183. https://doi.org/10.1186/s12911-022-01923-5
Hi Marcia, well done! Your use case is very thorough and addresses an important research need.
It will be interesting to see the similarities and differences between the NLP thematic analysis and researcher thematic analysis. One issue I can think of is that the researcher has an understanding of the context of the research question and may identify themes as more or less important accordingly. The NLP does not have that – is that a strength or weakness? Who knows!
The applications of a systematized interview coding system that leverages AI sounds so incredibly efficient. As someone that has spent some time coding interviews for qualitative research, this sounds incredibly time-saving. I am excited to see how this could positively affect the field of research.
Marcia this was a fantastic presentation and start to your project. I appreciated how you outlined the various models you would use in order to maximize researcher efficiency. It is completely true that we need more time to develop initiatives to drive policy changes and I think utilizing the resources differently will really help to achive this goal and drive change. I am curious if there is a specific topic that you would first like to explore? The idea of behavior change is so vast so I wonder where you would start?
Hi Marcia, exciting work! Your project demonstrates the potential to unlock new insights from larger datasets while reducing the labor intensity of manual coding, which could truly transform qualitative research, making it faster and more accessible!
Hi Marcia, I am very excited for your project! I feel that your work would be a game changer for researchers collecting qualitative interview data. I am curious about your thoughts on NLP and cultural competency/awareness of different dialects. Do you expect that NLP will be able to extract these nuances or will there need to be significant human oversight?
Marcia!! Processing qualitative research can be time-consuming and labor-intensive. It would be interesting to see how you can utilize AI for each behavior change theory and with different cultures. The individual narrative is critical to this work, and addressing potential biases from AI is essential. I am so excited about this potential research.
Hi Marcia, your presentation was so clear and well-organized. Your project to enhance qualitative health behavior data analysis using AI and NLP techniques is highly innovative. I’m wondering how you will ensure that your AI models effectively capture the subtle culture-related contexts in health behavior research.