Bayesian Spatiotemporal Analyses and the Public Health Data Warehouse
We developed a comprehensive, social-ecological model of fatal opioid-involved overdoses to guide us in the choice of factors to extract from the massive Massachusetts Public Health Data Warehouse (PHD) for use in our Bayesian spatiotemporal models. In these models, we are measuring the independent and synergistic effects of predictors of opioid-involved overdose deaths, assessing trends over time and across Massachusetts neighborhoods. The PHD is a unique resource with data gathered from more than 80 datasets and linked at the individual level. These data, safeguarded by multiple layers of privacy protection, include Medical Examiner records of fatal overdoses, ambulance records of nonfatal overdoses, health insurance claims, hospital records, and toxicology data. Still using robust privacy safeguards, we are linking datasets at the level of the individual and connecting them to community level data, including measures of the social determinants of health, such as race and poverty.
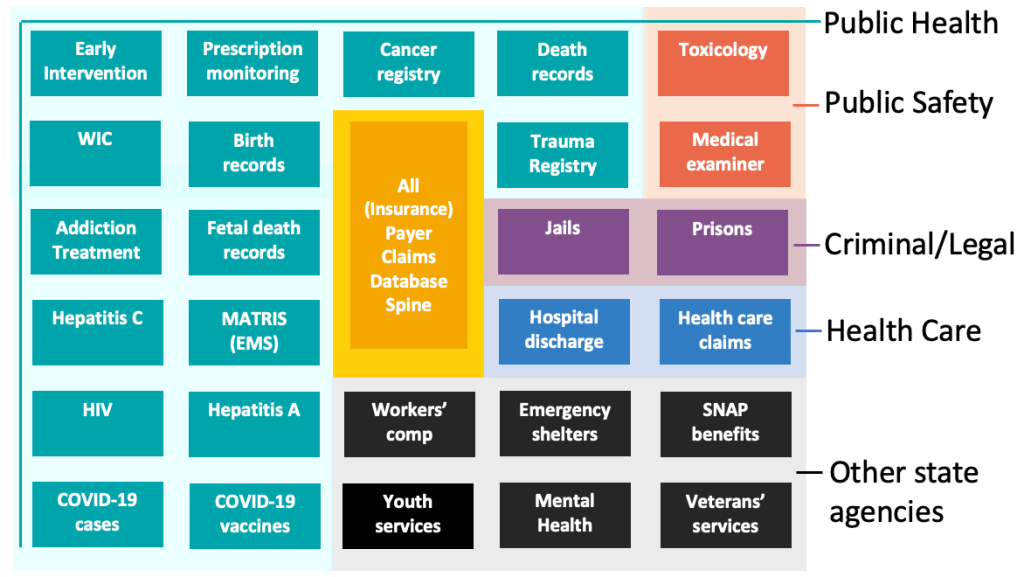
(MDPH, 2024)
Building on our improved understanding of the associations between these factors, opioid-involved overdoses, and fatal overdose hotspots in Massachusetts, we will construct a dynamic predictive model, forecasting opioid overdose counts and overdose hotspots and coldspots. Our goal is to assist in optimal resource allocation and place-based strategic planning. Our models will enhance surveillance efforts for the Massachusetts Department of Public Health, be replicable in other jurisdictions, and facilitate early detection of future overdose spikes to inform preemptive public health and harm reduction responses.
In 2023 we laid groundwork for our predictive models with the publication Small Area Forecasting of Opioid-Related Mortality: Bayesian Spatiotemporal Dynamic Modeling Approach*. From that paper in JMIR Public Health and Surveillance, here is a map of predicted fatal opioid-involved overdose mortality rates per 100,000 by ZIP Code in Massachusetts in 2021:

*Bauer, C., Zhang, K., Li, W., Bernson, D., Dammann, O., LaRochelle, M.R., Stopka, T.J., 2023. Small Area Forecasting of Opioid-Related Mortality: Bayesian Spatiotemporal Dynamic Modeling Approach. JMIR Public Health and Surveillance 9, e41450. DOI: 10.2196/41450.