Group publishes paper showing convolutional neural networks working on real LArTPC data for the first time
Our groups’ work, done in collaboration with colleages Kazu Terao (SLAC) and Vic Genty (Columbia), was posted onto the arxiv recently, titled A Deep Neural Network for Pixel-Level Electromagnetic Particle Identification in the MicroBooNE Liquid Argon Time Projection Chamber
The article describes a convolutional neural network (CNN) that labels track and shower pixels.
Importantly, it was the first time results were shown for a CNN working on real data from a liquid argon TPC, the detector employed by several current and future neutrino experiments. By comparing how a human analyst and the network labeled both simulated and real data, the paper demonstrates that these networks can work similarly on both — even though the networks were trained on simulated data alone. This is an important demonstration for the community to trust physics results coming from analyses which use the outputs from these networks.
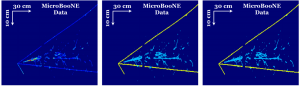
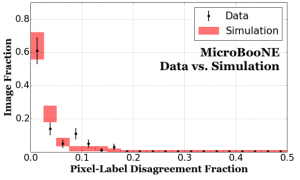