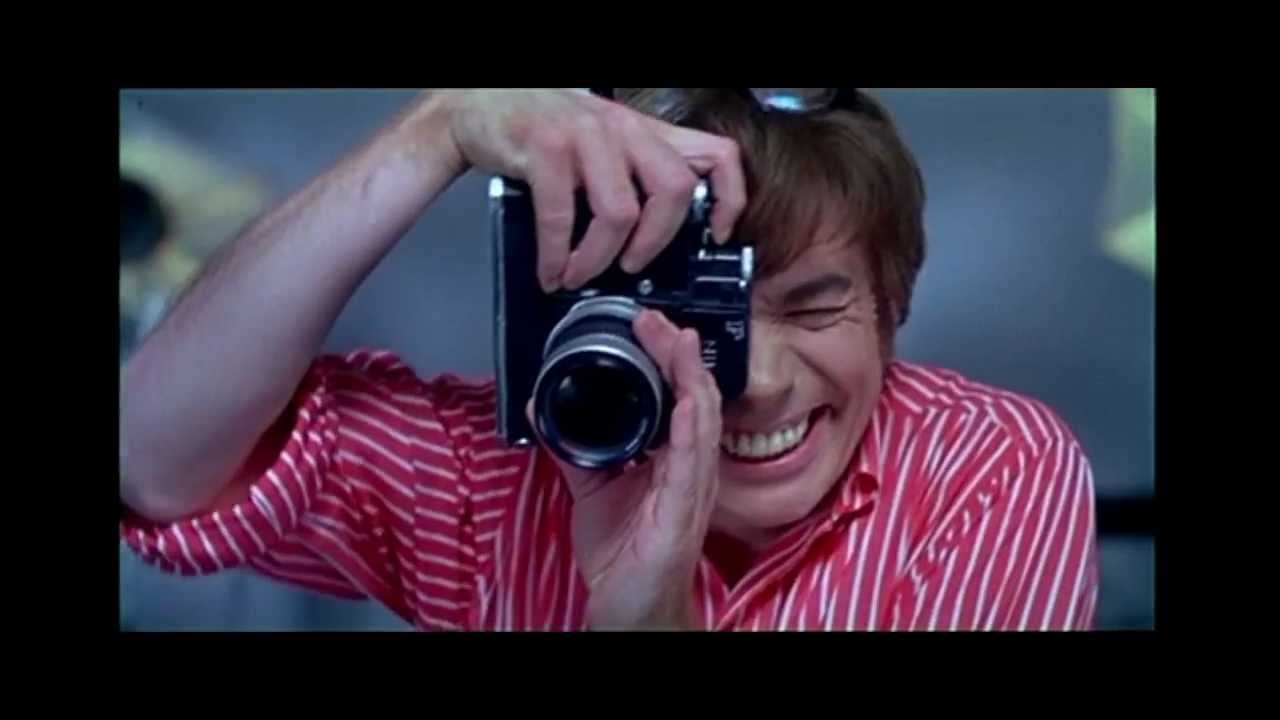
If you had the day off tomorrow to do whatever you want, what would you do? The answer to this question depends entirely on who you ask. But are there similarities between the mental mechanisms everyone uses to make this choice? When a decision like this relies on one’s intrinsic value of activities, the […]