Hybrid Beamforming Design Problem in WideBand Wireless Communication Systems
Project Overview:
The current trend of new technologies such as autonomous driving and the Internet of Things (IoT) has generated a growing demand for higher data transmission rates. This demand can be met by leveraging the THz communication network. However, the large bandwidth in the THz spectrum may cause a challenging effect, which is known as the “beam squinting problem”, which can lead to severe performance degradation. In this project, we are seeking solutions to this issue within the context of a hybrid beamforming design problem.
Hybrid beamforming, which is a combination of digital and analog beamforming techniques, can effectively decrease the implementation cost but also increase the data rate, compared with purely digital or analog beamforming design.
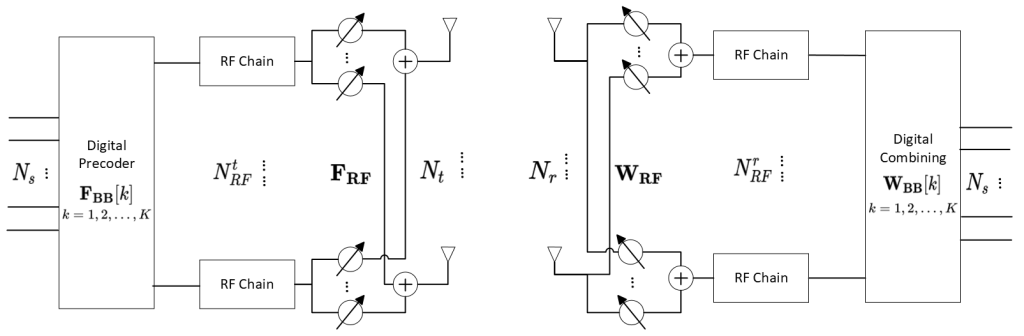
Background and Motivation:
Analog beamforming with phased arrays is a promising and widely used 5G wireless communication system technology. The beamforming is used for directional signal transmission and reception, and by using this technology, we can adjust the phases of the antenna elements by using the phase shifters (PSs) to steer the beams in certain directions.
Usually, when the signal arrives (or departs), there will be an angle between the signal and the antennas, leading to time discrepancies among different antennas. The idea to address this issue is to use the PSs to make the arrived signals get the same phase. Due to the practical hardware constraint, we use the carrier frequency as the specific frequency of all the RF phase shifters in a phased array. However, as we discussed above, for wideband systems, the array gain will become frequency-dependent, which causes the beam squinting problem.

Methods:
So far, we’ve tried two approaches to address this problem. First, we’ve formulated an optimization problem and used traditional numerical algorithms to find the solutions. Because of the nonconvex nature of the problem, which presents the complexities, we’ve been exploring specialized techniques like manifold optimization to address it effectively. The second method is to construct a Bipartite Graph Neural Network (BGNN) model to deal with this problem. We use 2 different kinds of nodes to represent the analog beamformers and digital beamformers separately, then use the message-passing model to update the nodes representations.