Hybrid Reconfigurable Intelligent Surface
Project overview:
The future networks are expected to support massive number of broadband devices seamlessly which requires the wireless system to meet unprecedently high throughput not achievable by current wireless standards. Solutions like mmWave and THz promise to achieve gigabit even terabit per second links, however, such high frequencies induce major coverage and connectivity challenges.
Reconfigurable intelligent meta-surface (RIS) is a promising solution to tackle these high frequency challenges. They are envisioned to assist transceivers enhancing the environment through intelligently reflecting and shaping the signals. These surfaces can be strategically placed indoors (e.g., on walls and ceiling) or outdoors (e.g., on building surfaces, billboards), enabling smart radio environment.
Background and Motivation:
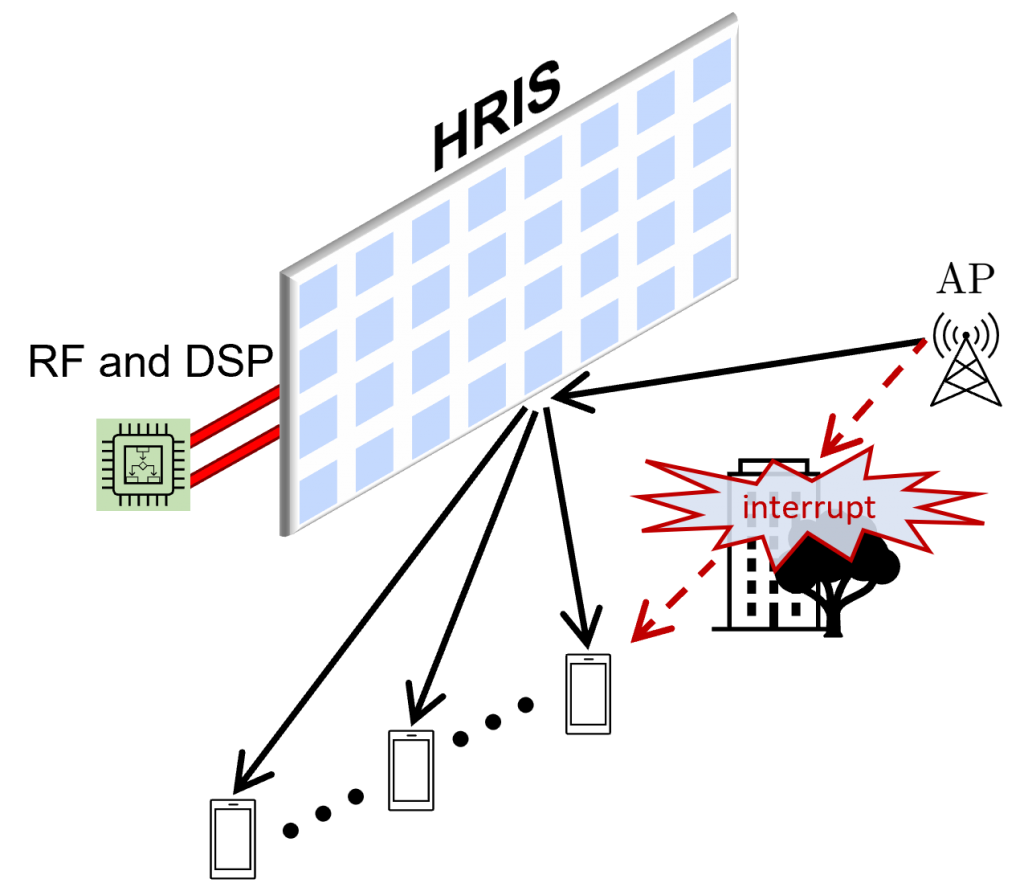
The most considered RIS is a passive reflector unable to receive signals, which unveils only a portion of the potentials of RIS. Moreover, such passive reflector use introduces major limitations in channel estimation and transmission scheme design along with restrictions in network management.
Therefore, our research focuses on merging RIS reflection ability with additional reception and transmission capabilities, called Hybrid RIS (HRIS). Commonly considered HRIS has a few active elements, each connected to a separate RF port. However, recent studies demonstrate the feasibility of designing meta-surfaces with RFs ports where each RF collects signals from multiple meta-elements, reducing cost, profile, and power consumption. These advantages enable the use of multiple HRISs to facilitate ubiquitous coverage for reliable real-time applications.
Methods:
To employ the HRISs and achieve high data rates, we propose novel algorithms which solve the fundamental challenges including accurate channel estimation and beamforming.
To achieve accurate channel estimation at the HRIS with only a few available RF ports, we propose two novel compressive sensing (CS) based channel estimation approaches. The proposed approaches jointly estimate the channels between multiple multi- antenna devices and the HRIS using only short pilot sequences, without requiring orthogonal pilots. The first approach uses CS to estimate both the angle and complex gain of each wireless propagation path, whereas the second approach uses CS to estimate the path angles then least square method to estimate the path gains.
To optimize reflection through the HRIS and enable efficient beamforming of the transmitter, we also employ state-of-the-art AI techniques and extensively study graph neural networks (GNN). The GNN approach we utilize empowers the HRIS to learn and adapt its reflection coefficients based on the surrounding environment. Additionally, it allows the transmitter to learn how to shape its beams effectively, ultimately leading to significant improvements in throughput performance.
Results:
Simulation results show that our channel estimation algorithms achieve high accuracy, are very robust to system settings (such as number of HRIS elements, users’ antennas, and number of users) and that more accurate channel estimates improve the spectral efficiency.
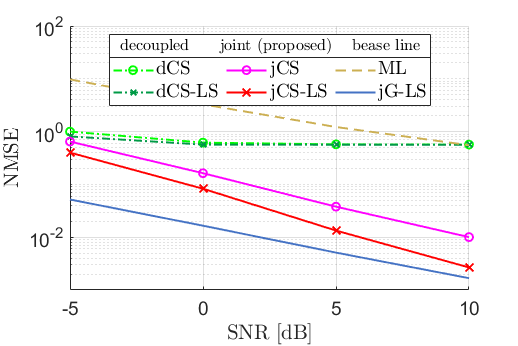
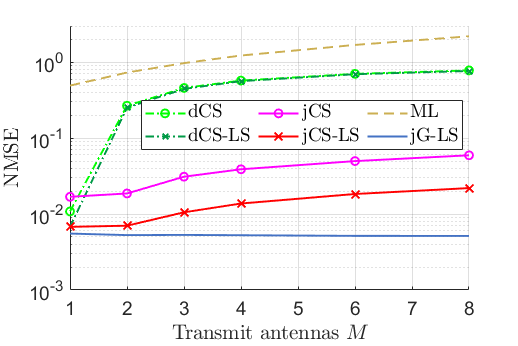
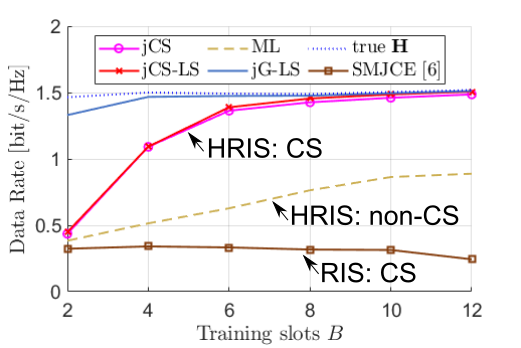
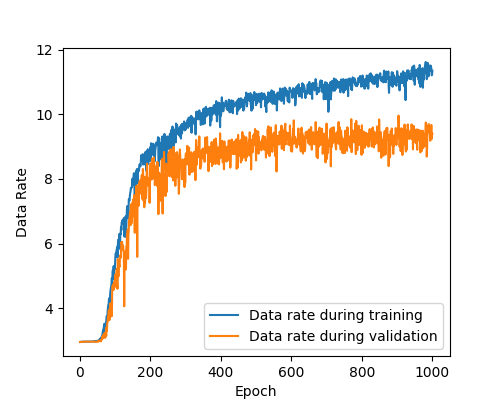
Simulation results also confirm that appropriately modeled GNNs have the capability to learn HRIS reflection and transmitter beamforming, even in the presence of multiple receivers.
Publication:
- “Joint Multi-User Channel Estimation for Hybrid Reconfigurable Intelligent Surfaces,”
B. Boiadjieva and M. Vu, IEEE Int’l Conf. on Communications (ICC), May 2023.
Acknowledgment:
This work was supported in part by the National Science Foundation under HDR TRIPODS Grant 1934553 and CNS Grant 1908552.