Mobile Edge Computation Offloading and Wireless Power Transfer
Mobile Edge Computation Offloading and Wireless Power Transfer
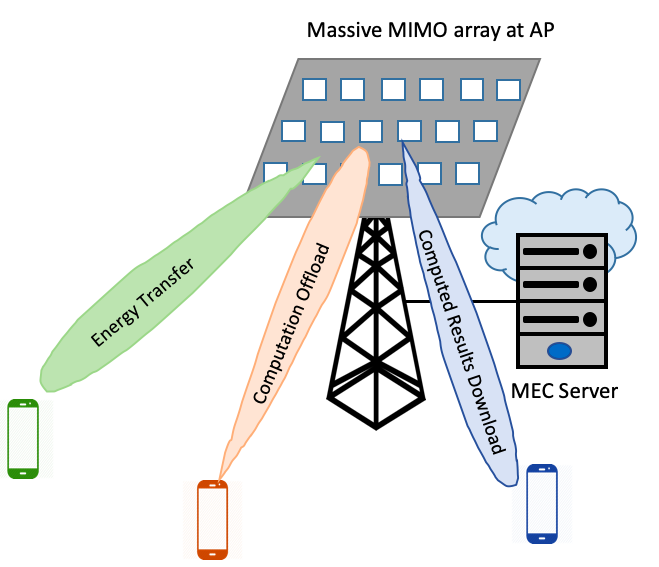
Project Overview:
Wireless communication networks have evolved towards denser deployments with an increasingly large number of connected devices. Future generation networks including 5G and beyond are therefore expected to offer services at high data rate and ultra-low latency. To address this challenge, Multi-access Edge Computing (MEC) is a promising technology which can provide distributed and decentralized services in close proximity to mobile subscribers at low latency, and high rate access. In this project we work on a resource allocation problem for system level energy minimization in a network where multiple Access Points (APs) with integrated edge servers are equipped with massive MIMO antenna arrays. The MEC-APs simultaneously accommodate multiple co-channel users and provide computation offloading and wireless charging to ground users.
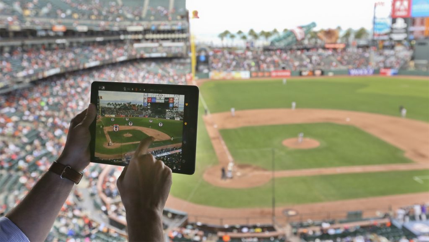
Motivation:
There has been a recent trend towards data-intensive services, for example, Augmented/Virtual Reality (AR/VR), autonomous vehicles, and smart cities (IoT). Such services need power-hungry designs and computation intensive features which is infeasible with limited battery and processing power of most consumer devices. Cloud computing capabilities provided by multi-access edge computing with proximate access can address this challenge and prove as a key technology in next generation networks. An example scenario where computation offloading could be used is in a crowded stadium, where service providers use AR/VR technology to provide a real-time experience to their viewers by capturing data from multiple surrounding cameras. This computationally taxing and latency-critical task of providing an enjoyable experience to the users could only be possible through edge computing.

Resource Allocation in MEC Networks:
Our goal is to explore the benefits of massive MIMO transmission, wireless power transfer and computation offloading for system level energy minimization. We consider an optimization problem for minimization of the weighted sum of energy consumed at the user and MEC subject to a latency requirement, constraints on power and CPU processing capabilities at the users and the MEC. We divide the system functions into three phases: (i) data offloading from the users to the MEC in uplink, (ii) wireless charging, computation at the MEC server and locally at the user, and (iii) downloading of computed results from MEC to users. Through a nested algorithm architecture we find the optimal data partition for computation offloading, and the optimal time and frequency allocation.
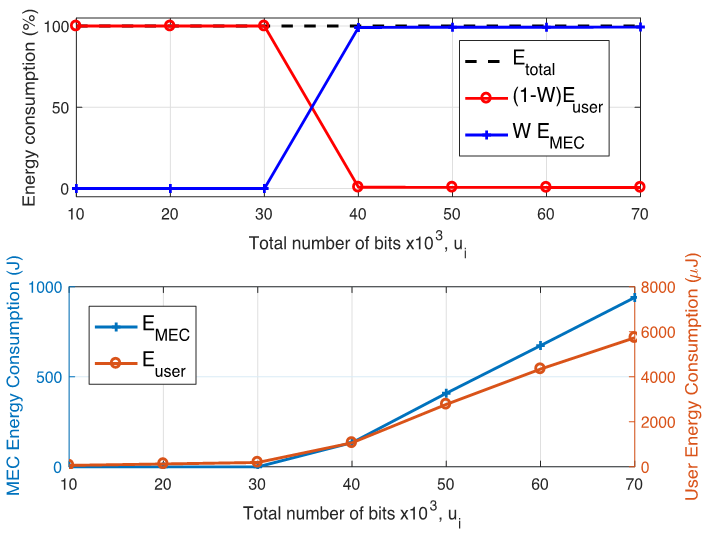
Results:
The energy consumption is proportional to the amount of data requested. For low data requests, all data is computed locally and the energy consumption is only for users’ computation. But for higher data requests, more than half the data is offloaded and the MEC’s energy consumption becomes dominant.
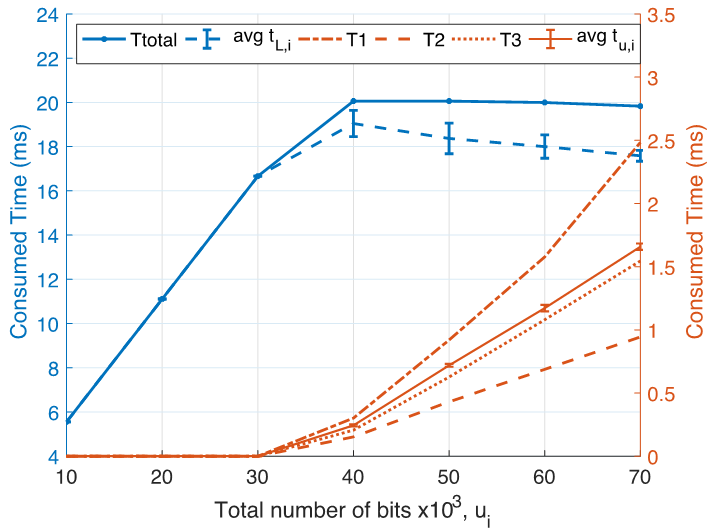
We observe that the time for local computation () at users is dominant due to their lower CPU frequencies. The time for wireless transmission is higher than the time for computation at the MEC, since the MEC’s high CPU frequency allows for fast processing. As expected, the time for offloading in the first phase is greater than the download time in the third phase due to the difference in user and AP transmit powers